The Unstructured Data Opportunity
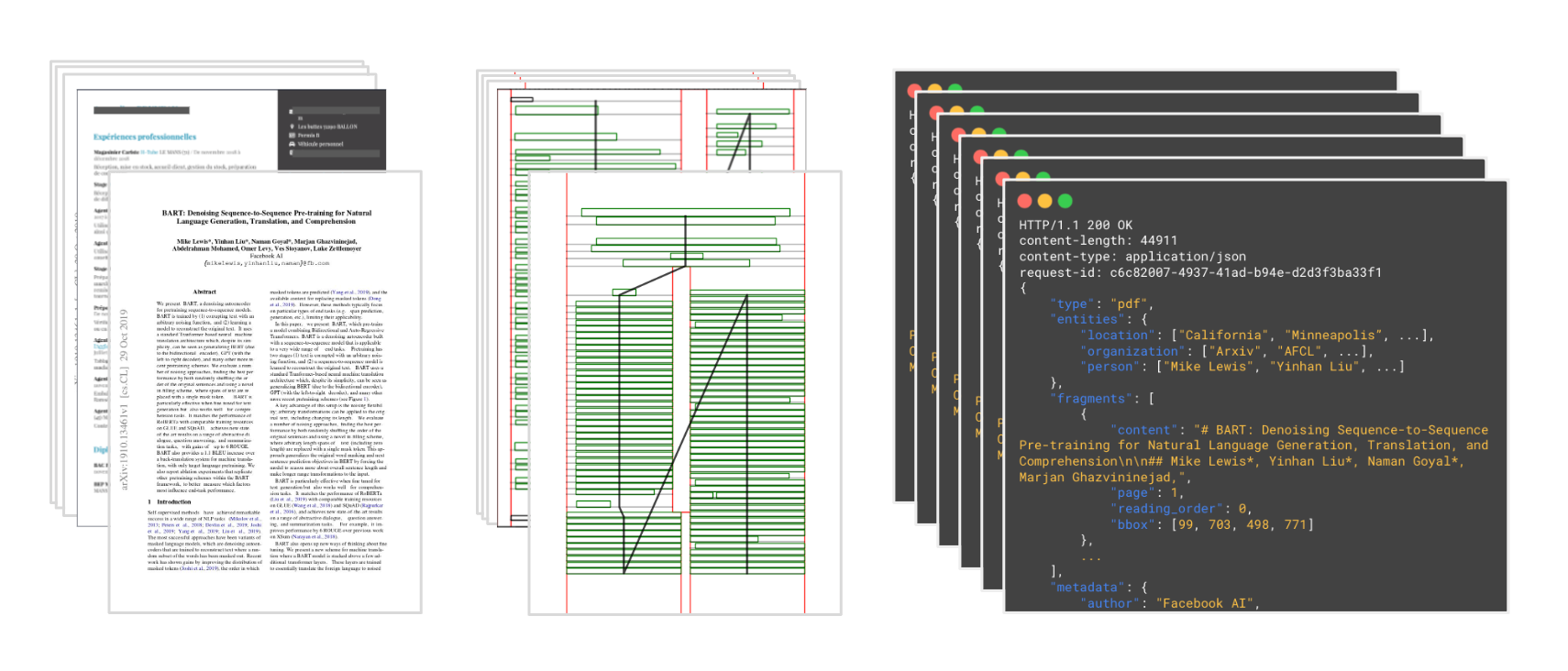
In today’s fast-evolving digital landscape, unstructured data represents one of the greatest untapped opportunities for businesses. For professional services companies especially—spanning finance, legal, banking, insurance, and more—capitalising on unstructured data workflows is not just a path to competitive advantage but a means to survival in an increasingly AI-driven market. Organisations can now deliver better services at lower costs, but the road to unlocking this value is far from straightforward. Entropia is here to help.
80% of corporate data is unstructured, leaving many workflows un-automated
Unstructured data refers to information that doesn’t fit neatly into traditional data models like spreadsheets or databases. Think of emails, legal contracts, PDFs, Presentations, Memos, customer service chat logs, meeting minutes, social media posts, …. Unlike structured data, which is easily searchable and organised, unstructured data lacks a predefined format, making it harder to analyse and integrate into automated workflows.
Though 80% of all corporate data is unstructured, most automations have remained focused on the structured, leaving much of this rich source of information underutilised. Well, at least until now.
Automating Unstructured Data workflows is difficult
Automation runs into three main barriers when it comes to unstructured data :
- Cost-effective pre-processing: Before any meaningful analysis can take place, the data must be prepared—sorted, cleansed, and standardised into a format that downstream AI models can digest. This process is both time-consuming and resource-intensive, because of the variety of document formats, which all require specific techniques to be orchestrated, and because of the sheer volume of data to process in parallel. Poor pre-processing leads to inconsistent results, making the effort of automation less reliable. "garbage-in, gargbage-out"
- Cost-effective foundational models: Current AI models can analyze unstructured data, but balancing accuracy and affordability is no easy feat. High-precision models can be prohibitively expensive, while cheaper alternatives may not deliver the required accuracy.
- User control : For companies, automating data processes without the ability to inject bespoke business logic into the analysis is a non-starter. Whether it’s interpreting a contract in a legal case or assessing a financial audit, firms need to ensure AI respects the nuances of their workflows. Ensuring users have sufficient control without undermining efficiency is critical.
Advances in Natural Language Processing are lowering the barriers
The challenges are formidable, but AI is rapidly evolving, and two key trends in natural language processing (NLP) are lowering the barriers to automating unstructured data workflows, making cost-effective foundational models a reality:
- The value per token is increasing : Thanks to improvements in model architectures and the quality of training data, each unit of processed data—each “token”—yields more insightful, higher-quality outputs.
- The price per token is falling : Advances in hardware and the development of steerable AI models are driving down the cost of running model inference.
These dynamic significantly enhances the value of investing in unstructured data pre-processing, as the resulting downstream applications are becoming increasingly powerful and valuable.
Open-source models are especially suitable for enterprise use
Open-source large language models (LLMs) have closed the gap with their closed-source counterparts, thanks to advancements in community-driven development and access to cutting-edge research1. Once seen as inferior in both performance and innovation, open-source LLMs are now competing at the highest levels, offering comparable accuracy, speed, and versatility.
For companies, this shift presents significant advantages. Open-source models can dramatically improve service quality while reducing costs. Thye can be customised and fine-tuned to address specific business needs and data, resulting in better performance and relevance. Their size for a given task can be optimised, reducing the inference infrastructure bill. Companies can integrate them into existing systems without the restrictions often imposed by proprietary solutions, and without worrying about scaling costs tied to per-user pricing typically found in proprietary solutions. Additionally, the transparency of open-source LLMs offers better insights into model behaviour, enhancing trust and security. Businesses can audit and understand exactly how the model works.
The opportunity to streamline unstructured data workflows is immense, especially for Professional Services companies
All companies can and will benefit from these new technologies, but the opportunity is especially transformative for Professional Services. From law to finance to insurance to consulting or audit, the automation of unstructured data workflows will define the next decade.
Claims, contracts, market reports, policies, … professional services are heavily reliant on complex documentation. And suddenly, much of this complex material can be processed by machines. Just like financial companies have long been delegating calculations to their computers via Excel, those professions will soon have machines read and analyse complex documents, which will transform their capacities and cost structures.
In Legal, analysing vast amounts of case law, statutes, documents, emails, or legal precedents for legal research or e-discovery will take seconds rather than hours. In Finance, automatic analysis of financial statements, audits, and reports will free up hours of manual labor, enabling faster decision-making. Similarly, the insurance sector will see radical improvements in claims processing and fraud detection, with AI automating everything from policy renewals to underwriting. The list goes on.
While previous waves of technological innovation focused on streamlining structured data—such as financial records or client databases—the next wave will centre on unstructured data. Five years from now, the landscape of professional services will look dramatically different, with AI playing a pivotal role in how unstructured data is analysed, interpreted, and actioned. AI will allow professionals to focus on higher-value tasks, while also enhancing accuracy and speed. This shift will is about to reshape the competitive landscape in these knowledge-intensive sectors.
Entropia unlocks the potential of your unstructured data
Entropia sits at the heart of this transformation. Our solutions target the pain points that have long plagued unstructured data workflows, offering a range of tools designed to streamline processes. Here’s an overview of what we already have in store :
- Data quality : document pre-processing We transform messy, unstructured data into a format that AI can readily digest, and are able to do this at enterprise scale. This ensures that the downstream models work with clean, high-quality inputs, not only improving the reliability of the insights produced, but also reducing their usual cost.
- Document Search & Analysis
Our Documentalist can organise and classify a knowledge base, retrieve any information from within millions of files regardless of language, and analyse documents on the fly.
For example, one of our customers (a tax consulting firm) uses Documentalist to find answers to current inquiries in past cases. When a question is submitted, Documentalist will look up the firm’s shared drive, find all documents which deal with a similar issue, read them, and answer the questions while pointing the user to relevant source passages. Before Documentalist, this knowledge was buried in dropbox in the form of pdf and scans, and employees didn’t have a way to leverage the firm’s historical knowledge. Thanks to Documentalist, they produce better outputs much faster. - Structure unstructured data
Another very powerful thing that Entropia can do, is to enable users to generate structured insights from unstructured files. Because we are able to parse the content of files and analyse them on the fly, we can perform multiple analysis on multiple documents simultaneously.
For instance, we collaborate with surgeons on a medical research project. They had envisioned a solution to extract statistical patterns from their patient records, but the data was trapped in PDFs and unstructured text, halting their progress. We helped by converting the PDFs into a structured text format and automatically generating table views from the text index—eliminating the need for manual data entry, and accelerating medical research. - Automate workflows
Every company has unique processes, taxonomies, and data structures that set it apart. Off-the-shelf solutions often fall short in addressing the unique challenges and nuances of a company’s workflows. This is why go beyond by helping companies automate specific, repetitive tasks, boosting efficiency and reducing errors.
Our technologies are versatile and adaptable, allowing us to tailor solutions to fit various industries and needs. We integrate seamlessly with existing systems and accommodate unique data structures to meet each client’s goals. We also develop no-UI (no User Interface) products that simplify testing, deployment, and operation. By removing unnecessary complexity, our solutions integrate easily into workflows, enabling quick adoption and immediate productivity gains.
Footnotes
- This summer Meta released Llama 3.1, and its performance on most established benchmarks reinforced the trend: closed-source models hold only a slight edge over open-source ones, and that gap typically takes just a few months for the open-source developer community to close - as captured in the following graph :
↩